C. A. Rivera-Romero | Universidad Autónoma de Zacatecas |
J. U. Muñoz-Minjares | Universidad Autónoma de Zacatecas |
R. Olivera-Reyna | Universidad Autónoma de Zacatecas |
E. R. Palacios-Hernandez | Universidad Autónoma de San Luis Potosí |
I. A. Reyes-Portillo | Universidad Autónoma de San Luis Potosí |
https://doi.org/10.58571/CNCA.AMCA.2022.023
Resumen: Spectral signature analysis is one of the most widely used techniques for diagnosing diseased plants. For this, it is necessary to consider different techniques for the feature extraction that allow the identification of different damage levels of a specific pathology, such as the case of fungal disease in cucurbits plants. In this study, reflectance measurements of healthy and diseased leaves are used to identify three main stages of powdery mildew levels: leaves in the germination stage of the fungus leaves with first symptoms and diseased leaves. Then, a proposal to use frequency analysis of the spectral signatures using the Wavelet transforms and the Fourier transforms to the feature extraction from the obtained coefficients and determines the damage levels using multi-classification in support vector machine blocks. Classification accuracy of 94.6% and 98.3%, respectively, was demonstrated. Therefore, this methodology is important for the diagnosis of damage levels on cucurbits leaves and other similar plants.
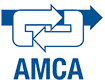
¿Cómo citar?
C. A. Rivera-Romero, E. R. Palacios-Hernandez, J. U. Muñoz-Minjares, R. Olivera-Reyna & I. A. Reyes-Portillo. Diagnosis in cucurbits plants based on Wavelet and Fourier coefficients using support vector machines. Memorias del Congreso Nacional de Control Automático, pp. 73-78, 2022. https://doi.org/10.58571/CNCA.AMCA.2022.023
Palabras clave
Otros Tópicos Afines; Redes Neuronales; Modelado e Identificación de Sistemas
Referencias
- Bajwa, S., Rupe, J., y Mason, J. (2017). Soybean disease monitoring with leaf reflectance. 9, 127.
- Denstedt, M., Bjorgan, A., Milanic, M., y Randeberg, L.L. (2014). Wavelet based feature extraction and visualization in hyperspectral tissue characterization. Biomedical Optics Express, 5.
- Feng, S., Duarte, M.F., y Parente, M. (2015). Universality of wavelet-based non-homogeneous hidden markov chain model features for hyperspectral signatures. In 2015 IEEE Conference on Computer Vision and Pattern Recognition Workshops (CVPRW), 19–27.
- Kempeneers, P., De Backer, S., Debruyn, W., Coppin, P., y Scheunders, P. (2005). Generic wavelet-based hyperspectral classification applied to vegetation stress detection. IEEE Transactions on Geoscience and Remote Sensing, 43(3), 610–614.
- Kumar, A. y Prema, P. (2015). A study on weed discrimination through wavelet transform, texture feature extraction and classification. International Journal of Computer Science and Information Technology, 7.
- Kuska, M.T., Bohnenkamp, D., Brugger, A., Alisaac, E., Wahabzada, M., Behmann, J., y Mahlein, A.K. (2018). Benefits of hyperspectral imaging for plant disease detection and plant protection: a technical perspective. Journal of Plant Diseases and Protection, 125(5), 5–20.
- Leonarduzzi, R., Schlotthauer, G., y Torres, M.E. (2010). Wavelet leader based multifractal analysis of heart rate variability during myocardial ischaemia. IEEE Engineering in Medicine and Biology Society, 2010, 110–3.
- Li, T. y Zhou, M. (2016). Ecg classification using wavelet packet entropy and random forests. Entropy, 18, 285.
- Luo, J., Huang, W., Zhao, J., Zhang, J., Zhao, C., y Ma, R. (2013). Detecting aphid density of winter wheat leaf using hyperspectral measurements. Selected Topics in Applied Earth Observations and Remote Sensing, IEEE Journal, 6, 690–698.
- Raju, A., Veni, S., y Jagatheesan, A. (2021). Robust classification technique for hyperspectral images based on 3d-discrete wavelet transform. Remote Sensing, 13, 1255.
- Rivera-Romero, C.A., Palacios-Hernández, E.R., Morales- Saldaña, J.A., y Olivera-Reyna, R. (2021). Feature extraction of powdery mildew levels in cucurbits leaves using wavelet-based and Fourier transforms. In 2021 IEEE International Autumn Meeting on Power, Electronics and Computing (ROPEC), volume 5, 1–6.
- Rivera-Romero, C.A., Palacios-Hernández, E.R., Trejo-Durán, M., Olivera-Reyna, R., y Morales-Saldana, J.A. (2020). Visible and near-infrared spectroscopy for detection of powdery mildew in Cucurbita pepo L. leaves. 14(4), 1–19.
- Rumpf, T., Mahlein, A.K., Steiner, U., Oerke, E.C., Dehne, H.W., y Pl¨umer, L. (2010). Early detection and classification of plant diseases with support vector machines based on hyperspectral reflectance. Computers and Electronics in Agriculture, 74(1), 91– 99.
- West, T., Prasad, S., y Bruce, L.M. (2007). Multiclassifiers and decision fusion in the wavelet domain for exploitation of hyperspectral data. In 2007 IEEE International Geoscience and Remote Sensing Symposium, 4850–4853.