Gil, Algemiro J. | Universidad de Guanajuato |
Torres Zúñiga, Ixbalank | Universidad de Guanajuato |
https://doi.org/10.58571/CNCA.AMCA.2024.076
Resumen: In this article, we explore an analytical approach to generate Takagi-Sugeno (TS) type models from ’experimental’ data using the Sparse Identification of Nonlinear Dynamics (SINDy) technique. Our method combines the approximation of reduced nonlinear models with the linearization of the same model at specific operating points, allowing us to capture the dynamics of complex systems under variable conditions for each point of interest. We then present a detailed implementation of this approach, highlighting its application in a real-world scenario of modeling a biotechnological process.
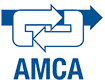
¿Cómo citar?
Gil, A.J. & Torres, I. (2024). Data-Driven Modeling of a Bioreactor Using a Takagi-Sugeno Type Approach.. Memorias del Congreso Nacional de Control Automático 2024, pp. 445-450. https://doi.org/10.58571/CNCA.AMCA.2024.076
Palabras clave
Dynamic systems modeling, Polytopic models, Sparse Identification, Bioreactor
Referencias
- K. Mehran. Takagi-Sugeno Fuzzy Modeling for Process Control. Industrial Automation, Robotics and Artificial Intelligence, School of Electrical, Electronic and Computer Engineering, Newcastle University, 2008.
- M. Bernal, A. Sala, Z. Lendek and T.M. Guerra. Analysis and Synthesis of Nonlinear Control Systems: A Convex Optimisation Approach. Studies in Systems, Decision and Control, 408, Springer, 2022.
- M. Abyad, A. Karama and A. Khallouq. Modelling and Control of a Biological Process Using the Fuzzy Logic Takagi -Sugeno. International Renewable and Sustainable Energy Conference (IRSEC), 1-5, 2017.
- C.H. Tinh, V.H. Tien, N.C. Dinh and M.N. Anh. Design of Takagi-Sugeno fuzzy controller for automatic stabilization system of missiles with blended aerodynamic and lateral impulsive reaction-jet. IEEE Symposium on Computational Intelligence for Security and Defense Applications (CISDA), 2014.
- H.H. Goh, J. Kang, D. Zhang, H. Liu, W. Dai, T. A. Kurniawan and K.C. Goh. Improving the Performance of DC Microgrids by Utilizing Adaptive Takagi-Sugeno Model Predictive Control. CSEE Journal of Power and Energy Systems,vol. 9, 4:1472-1481, 2023.
- K.B. Ariyur and M. Krstic. Real-Time Optimization by Extremum-Seeking Control. Adaptive control systems. John Wiley and Sons, Inc., Hoboken, New Jersey, 2003.
- S.L. Brunton, J.L. Proctor, and J.N. Kutz. Discovering governing equations from data by sparse identification of nonlinear dynamical systems. Princeton University, Princeto, 2015.
- S.H. Rudy, S.L. Brunton, J.L. Proctor, and J.N. Kutz. Data-driven discovery of partial differential equations. Science Advances 3.4, 2017.