Pérez-Pérez, Esvan | Instituto Tecnológico de Tuxtla |
López-Estrada, F. R. | Instituto Tecnológico de Tuxtla |
Vicenç Puig | Institut de Robòtica iInformàtica Industrial |
Resumen: This work proposes a method to diagnose faults in a wind turbine. The proposed approach combines the use of Analytical Redundancy Relationships (ARR) and an Artificial Neural Network (ANN). The ARR is used to generate a matrix of fault signatures through structural analysis, while the ANN is used to classify the detected faults. The results of the diagnostic system are presented using the measurements obtained from the model of a 4.8 [MW] wind turbine.
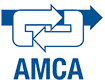
¿Cómo citar?
Esvan de Perez,Francisco Ronay López-Estrada & Vicenç Puig. Diagnosis of faults in a wind turbine using Analytical Redundancy Relations and an Artificial Neural Network. Memorias del Congreso Nacional de Control Automático, pp. 1-6, 2020.
Palabras clave
Fault Diagnosis, Analytical Redundancy Relationships, Wind Turbines, Artificial Neural Networks, Data-based methods
Referencias
- Aguilar, C.Z., Gómez-Aguilar, J., Alvarado-Martínez, V., and Romero-Ugalde, H. (2020). Fractional order neural networks for system identification. Chaos, Solitons & Fractals, 130, 109444.
- Antoniadou, I., Dervilis, N., Papatheou, E., Maguire, A., and Worden, K. (2015). Aspects of structural health and condition monitoring of offshore wind turbines. Philosophical Transactions of the Royal Society A: Mathematical, Physical and Engineering Sciences, 373(2035), 20140075.
- Artigao, E., Martín-Martínez, S., Honrubia-Escribano, A., and Gómez-Lázaro, E. (2018). Wind turbine reliability: A comprehensive review towards effective condition monitoring development. Applied energy, 228, 1569–1583.
- Blesa, J., Puig, V., Romera, J., and Saludes, J. (2011). Fault diagnosis of wind turbines using a setmembership approach. In Proceedings of IFAC world congress, 8316–8321.
- Dey, S., Perez, H.E., and Moura, S.J. (2019). Robust fault detection of a class of uncertain linear parabolic pdes. Automatica, 107, 502–510.
- Habibi, H., Howard, I., and Simani, S. (2018). Reliability improvement of wind turbine power generation using model-based fault detection and fault tolerant control: A review. Renewable energy.
- Hameed, Z., Hong, Y., Cho, Y., Ahn, S., and Song, C. (2009). Condition monitoring and fault detection of wind turbines and related algorithms: A review. Renewable and Sustainable energy reviews, 13(1), 1–39.
- Hossain, M., Abu-Siada, A., and Muyeen, S. (2018). Methods for advanced wind turbine condition monitoring and early diagnosis: A literature review. Energies, 11(5), 1309.
- Leahy, K., Hu, R.L., Konstantakopoulos, I.C., Spanos, C.J., Agogino, A.M., and O’Sullivan, D.T. (2018). Diagnosing and predicting wind turbine faults from scada data using support vector machines. International Journal of Prognostics and Health Management, 9(1), 1–11.
- Lucas, F., Costa, P., Batalha, R., and Leite, D. (2018). High impedance fault detection in time-varying distributed generation systems using adaptive neural networks. In 2018 International Joint Conference on Neural Networks (IJCNN), 1–8. IEEE.
- Mokhtari, A. and Belkheiri, M. (2018). Fault diagnosis of a wind turbine benchmark via statistical and support vector machine. In International Journal of Engineering Research in Africa, volume 37, 29–42. Trans Tech Publ.
- Odgaard, P.F., Stoustrup, J., and Kinnaert, M. (2013). Fault-tolerant control of wind turbines: A benchmark model. IEEE Transactions on Control Systems Technology, 21(4), 1168–1182.
- Puig, V., Quevedo, J., Escobet, T., Morcego, B., and Ocampo, C. (2010). Control tolerante a fallos (parte i): Fundamentos y diagnóstico de fallos. Revista Iberoamericana de automática e informática industrial, 1(1), 15–31.
- Quiñones-Grueiro, M., Prieto-Moreno, A., Verde, C., and Llanes-Santiago, O. (2019). Data-driven monitoring of multimode continuous processes: A review. Chemometrics and Intelligent Laboratory Systems.
- Rezamand, M., Kordestani, M., Carriveau, R., Ting, D.S., and Saif, M. (2019). A new hybrid fault detection method for wind turbine blades using recursive pca and wavelet-based pdf. IEEE Sensors Journal.
- Sanchez, H., Escobet, T., Puig, V., and Odgaard, P.F. (2015). Fault diagnosis of an advanced wind turbine benchmark using interval-based arrs and observers. IEEE Transactions on Industrial Electronics, 62(6), 3783–3793.
- Tornil-Sin, S., Ocampo-Martinez, C., Puig, V., and Escobet, T. (2013). Robust fault diagnosis of nonlinear systems using interval constraint satisfaction and analytical redundancy relations. IEEE Transactions on Systems, Man, and Cybernetics: Systems, 44(1), 18–29.
- Ugalde, H.M.R., Carmona, J.C., Reyes-Reyes, J., Alvarado, V.M., and Mantilla, J. (2015). Computational cost improvement of neural network models in black box nonlinear system identification. Neurocomputing, 166, 96–108.
- Villanueva, A. and Verde, C. (2018). Fault diagnosis for descriptor systems by equivalent transformations. IFAC-PapersOnLine, 51(24), 1239–1246.
- Wang, H., Lei, Z., Zhang, X., Zhou, B., and Peng, J. (2019). A review of deep learning for renewable energy forecasting. Energy Conversion and Management, 198, 111799.
- Wang, Y., Ma, X., and Qian, P. (2016). Wind turbine fault detection and identification through pca-based optimal variable selection. IEEE Transactions on Sustainable Energy, 9(4), 1627–1635.
- Wang, Y., Ma, X., and Qian, P. (2018). Wind turbine fault detection and identification through pca-based optimal variable selection. IEEE Transactions on Sustainable Energy, 9(4), 1627–1635.
- Watson, S., Moro, A., Reis, V., Baniotopoulos, C., Barth, S., Bartoli, G., Bauer, F., Boelman, E., Bosse, D., Cherubini, A., et al. (2019). Future emerging technologies in the wind power sector: A european perspective. Renewable and Sustainable Energy Reviews, 113, 109270.
- Yang, H., Han, Q.L., Ge, X., Ding, L., Xu, Y., Jiang, B., and Zhou, D. (2019). Fault tolerant cooperative control of multi-agent systems: A survey of trends and methodologies. IEEE Transactions on Industrial Informatics.
- Yogitha, R. and Mathivanan, G. (2018). Performance analysis of transfer functions in an artificial neural network. In 2018 International Conference on Communication and Signal Processing (ICCSP), 0393–0397. IEEE.
- Yousefian, R. and Kamalasadan, S. (2017). A review of neural network based machine learning approaches for rotor angle stability control. arXiv preprint arXiv:1701.01214.