Guzmán Zaragoza Miguel Maximiliano | Centro Nacional de Investigacion y Desarrollo Tecnologico |
Jarniel García Morales | Centro Nacional de Investigacion y Desarrollo Tecnologico |
Carlos Daniel Garcia Beltran | Centro Nacional de Investigacion y Desarrollo Tecnologico |
Manuel Adam-Medina | Centro Nacional de Investigacion y Desarrollo Tecnologico |
Ricardo Fabricio Escobar-Jiménez | Centro Nacional de Investigacion y Desarrollo Tecnologico |
Cervantes-Bobadilla Marisol | Centro de Investigacion en Ingenieria y Ciencias Aplicadas |
Resumen: This work presents the fault detection and isolation (FDI) system design for the Throttle Position Sensor (TPS), Mass Air Flow (MAF), and Manifold Absolute Pressure (MAP) sensor of an internal combustion engine. The FDI system utilizes five multilayer perceptron artificial neural network (ANN), which were trained to estimate the value of each sensor using the crankshaft position (CKP) and the Air-Fuel ratio (AFR) sensors to generate analytical redundancy. When a fault is induced in one sensor, the FDI system replaces the faulty signal for an adequate estimation of the signal given by one ANN allowing uninterrupted operation of the internal combustion engine.
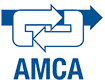
¿Cómo citar?
Guzmán Zaragoza Miguel Maximiliano, Jarniel García Morales, Carlos Daniel Garcia Beltran, Manuel Adam-Medina, Ricardo Fabricio Escobar-Jiménez & Cervantes-Bobadilla Marisol. Fault Detection and Isolation in Sensors of an Internal Combustion Engine. Memorias del Congreso Nacional de Control Automático, pp. 1-6, 2020.
Palabras clave
Fault Detection and Isolation, Internal Combustion Engine, Artificial Neural Networks
Referencias
- Amin, A.A. and Mahmood-ul Hasan, K. (2019). Robust active fault-tolerant control for internal combustion gas engine for air–fuel ratio control with statistical regression-based observer model. Measurement and Control.
- Carbot-Rojas, D., Besan¸con, G., and Escobar-Jim´enez, R. (2019). Ekf based sensor fault diagnosis for an internal combustion engine. In 2019 23rd International Conference on System Theory, Control and Computing (ICSTCC), 43 – 48. IEEE.
- Chen, J. and Patton, R. (1999). Robust Model-based Fault Diagnosis for Dynamic Systems. Kluger Academic Publisher.
- Escobar, R., Astorga-Zaragoza, C., Téllez-Anguiano, A., Ju´arez-Romero, D., Hernández, J.A., and Guerrero Ramírez, G. (2011). Sensor fault detection and isolation via high-gain observers: application to a double-pipe heat exchanger. ISA Trans., 50(3), 480–486.
- García-Morales, J., Adam-Medina, M., Escobar, R.F., Astorga-Zaragoza, C.M., and Garcia-Beltran, C.D. (2015). Diagnóstico de fallas múltiples en los sensores de un intercambiador de calor empleando observadores por modos deslizantes basado en el algoritmo supertwisting. Revista mexicana de ingeniería química, 14(2), 553–565.
- Guzzella, L. and Onder, C. (2009). Introduction to modeling and control of internal combustion engine systems. Springer Science & Business Media.
- Hendricks, E. and Luther, J.B. (2001). Model and observer based control of internal combustion engines. In Proceedings of International Workshop on Modeling, Emissions and Control in Automotive Engines (MECA01). Citeseer.
- Jung, D. (2020). Residual generation using physicallybased grey-box recurrent neural networks for engine fault diagnosis. arXiv preprint arXiv:2008.04644.
- León, P.G., García-Morales, J., Escobar-Jiménez, R.F., Gómez-Aguilar, J.F., López-López, G., and Torres, L. (2018). Implementation of a fault tolerant system for the internal combustion engine’s maf sensor. Measurement, 122, 91–99.
- Lin, T.H. and Wu, S.C. (2019). Sensor fault detection, isolation and reconstruction in nuclear power plants. Annals of Nuclear Energy, 126, 398–409.
- Mashadi, B. and Crolla, D.A. (2012). Vehicle powertrain systems. Wiley London.
- Meng, L., Luo, J., Yang, X., and Zeng, C. (2019). Intake air mass observer design based on extended kalman filter for air-fuel ratio control on si engine. Energies, 12(18), 34–44.
- Mu, W., Wang, J., and Feng, W. (2017). Fault detection and fault-tolerant control of actuators and sensors in distributed parameter systems. Journal of the Franklin Institute, 354(8), 3341–3363.
- Murtaza, G., Bhatti, A.I., and Butt, Y.A. (2018). Super twisting controller-based unified fdi and ftc scheme for air path of diesel engine using the certainty equivalence principle. Proceedings of the Institution of Mechanical Engineers, Part D: Journal of Automobile Engineering, 232(12), 1623–1633.
- Sangha, M.S., Gomm, J.B., Yu, D.L., and Page, G.F. (2005). Fault detection and identification of automotive engines using neural networks. IFAC Proceedings, 38(1), 272–277.
- Vasu, J.Z., Deb, A.K., and Mukhopadhyay, S. (2015). Mvem-based fault diagnosis of automotive engines using dempster–shafer theory and multiple hypotheses testing. IEEE Transactions on Systems, Man, and Cybernetics: Systems, 45(7), 977–989.
- Yu, D.L., Hamad, A., Gomm, J.B., and Sangha, M.S. (2014). Dynamic fault detection and isolation for automotive engine air path by independent neural network model. International Journal of Engine Research, 15(1), 87–100.