Galicia, Tonalli | Tecnológico Nacional de México, Tijuana |
Coria, Luis N. | Tecnológico Nacional de México, Tijuana |
Campos Hernandez, Paul Javier | Tecnológico Nacional de México, Tijuana |
Gamboa, Diana | Tecnológico Nacional de México, Tijuana |
https://doi.org/10.58571/CNCA.AMCA.2024.008
Resumen: This paper presents the design of an observer for a nonlinear model that describes the I and II Types of Diabetes Mellitus. The model includes a sigmoid function to represent the behavior of a discontinuous nonlinear function. Therefore, we give the conditions for the required gains for the observer’s convergence.
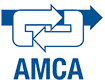
Observer for a Diabetes Mellitus mathematical model with a sigmoid function
Size: 387 KB
Total descargados hasta ahora
10 Downloads
¿Cómo citar?
Galicia, T., Coria, L.N., Campos, P.J. & Gamboa, D. (2024). Observer for a Diabetes Mellitus mathematical model with a sigmoid function. Memorias del Congreso Nacional de Control Automático 2024, pp. 43-48. https://doi.org/10.58571/CNCA.AMCA.2024.008
Palabras clave
Observer, Diabetes Mellitus, Sigmoid function
Referencias
- Agud Albesa, L. (2020). Método de bisección para la resolución de ecuaciones.
- Alzate Torres, C.M. (2022). Desarrollo de una estrategia de control predictivo no lineal y estimación de entradas desconocidas con aplicaciones a procesos biomédicos. Ph.D. thesis, Universidad Nacional de Colombia-Sede Medellín.
- Apablaza, P., Soto, N., and Codner, E. (2017). De la bomba de insulina y el monitoreo continuo de glucosa al páncreas artificial. Revista médica de Chile, 145(5), 630–640.
- Campos, P.J., Coria, L.N., and Trujillo, L. (2018). Nonlinear speed sensorless control of a surface-mounted pmsm based on a thau observer. Electrical Engineering, 100(1), 177–193.
- Cárdenas, M.O. (2009). Diseño de observadores con no linealidades fuertes. Ph.D. thesis, Universidad Nacional Autónoma de México.
- Castro-Gómez, J., Ramírez-Rasgado, F., Hernández-González, O., Astorga-Zaragoza, C., Valencia-Palomo, G., and Guerrero-Sánchez, M. (2022). Control for plasma glucose regulation in type 1 diabetes mellitus patients with unknown input delays.
- Copado, A.H. and Sánchez, E.C. (2019). Los sistemas digestivo y excretor (Valoración de la condición física e intervención en accidentes). Editex.
- Fregoso-Aparicio, L., Noguez, J., Montesinos, L., and García-García, J.A. (2021). Machine learning and deep learning predictive models for type 2 diabetes: a systematic review. Diabetology & metabolic syndrome, 13(1), 148.
- Gamboa, D., Coria, L., and Valle, P. (2022). Ultimate bounds for a diabetes mathematical model considering glucose homeostasis. axioms 2022, 11, 320.
- Islam, M.S., Qaraqe, M.K., Belhaouari, S.B., and Abdul-Ghani, M.A. (2020). Advanced techniques for predicting the future progression of type 2 diabetes. IEEE access, 8, 120537–120547.
- Khalil, H.K. (2002). Control of nonlinear systems. Prentice Hall, New York, NY.
- Krishchenko, A. (2005). Localization of invariant compact sets of dynamical systems. Differential Equations, 41(12).
- Lombarte, M., Lupo, M., Brenda, L.F., Campetelli, G., Marilia, A.B., Basualdo, M., and Rigalli, A. (2018). In vivo measurement of the rate constant of liver handling of glucose and glucose uptake by insulin-dependent tissues, using a mathematical model for glucose homeostasis in diabetic rats. Journal of Theoretical Biology, 439, 205–215.
- Lombarte, M., Lupo, M., Campetelli, G., Basualdo, M., and Rigalli, A. (2013). Mathematical model of glucose–insulin homeostasis in healthy rats. Mathematical biosciences, 245(2), 269–277.
- López, M.A.C., de Liger Serrano, J.L.F., Rodríguez, J.L.R., and Márquez, T.M. (2012). Validación de un glucómetro en una unidad de cuidados intensivos. Endocrinología y Nutrición, 59(1), 28–34.
- Lyapunov, A.M. (1992). The general problem of the stability of motion. International journal of control, 55(3), 531–534.
- Riihimaa, P. (2020). Impact of machine learning and feature selection on type 2 diabetes risk prediction. Journal of Medical Artificial Intelligence, 3.
- Rivadeneira Paz, P.S., Sereno, J.E., and Caicedo, M.A. (2019). Nuevas estrategias de control glucémico en pacientes con diabetes mellitus tipo 1.
- Stolfi, P., Valentini, I., Palumbo, M.C., Tieri, P., Grignolio, A., and Castiglione, F. (2020). Potential predictors of type-2 diabetes risk: machine learning, synthetic data and wearable health devices. BMC bioinformatics, 21, 1–19.