Diego M. Botín-Sanabria | Tecnológico de Monterrey |
Mauricio A. Ramíırez-Moreno | Tecnológico de Monterrey |
Jorge de J. Lozoya-Santos | Tecnológico de Monterreya |
https://doi.org/10.58571/CNCA.AMCA.2022.087
Resumen: Real-time pattern and activity recognition techniques have experienced a recent surge in the use of advanced statistical techniques for analyzing data. More explicitly, Machine Learning classification methods have been used in a variety of applications and for different purposes, such as recognizing different types of events from accelerometer data (e.g. in smart watches, wearables, and vibration analysis). The objective of this work is to present a novel use of accelerometer-based pattern recognition techniques to detect the urban space infrastructure characteristics based on their effect on a vehicles body frame dynamics. For this study, we focus on detecting speed bumps, potholes and curves based on a real-time data streaming with the objective of rendering a street digitalization to automatically update an urban space Digital Twin. The present study has achieved real-time event detection for an urban trajectory with high accuracy which yields data that can be later fused with other sensors data. This approach is effectively contributing to the infrastructure layer of a multi-layered approach of digital modelling. Furthermore, the results contribute to expand the body of knowledge of real-time accelerometer event recognition techniques.
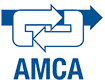
¿Cómo citar?
Botín-Sanabria, Diego M., Ramíırez-Moreno, Mauricio A. & Lozoya-Santos, Jorge de J. Real-time Accelerometer-Based Event Detection Through KNN Classification Method. Memorias del Congreso Nacional de Control Automático, pp. 545-551, 2022. https://doi.org/10.58571/CNCA.AMCA.2022.087
Palabras clave
Robótica y Mecatrónica; Detección y Aislamiento de Fallas
Referencias
- United Nations The Sustainable Development Goals Report 2020. UN Department of Economic and Social Affairs; 2020.
- MathWorks Inc. Estimating Orientation Using Inertial Sensor Fusion and MPU-9250. Matlab Documentation; 2022; R2022a.
- MathWorks Inc. Clasification learner. Matlab Documentation; 2022; R2022a.
- Zhang S.; Li X.; Zhong M.; Zhu X. & Wang R. Efficient kNN Classification with Different Numbers of Nearest Neighbors. IEEE Transactions on Neural Networks and Learning Systems, vol. 29; 2022.
- Farrahi V.; Niemela M.; Kangas M.; Korpelainen R. & Jamsa T. Calibration and validation of accelerometer based activity monitors: A systematic review of machine-learning approaches. Gait & Posture, vol. 68, pages 285-299; 2019.
- Miao J. & Niu L. A Survey on Feature Selection. Procedia Computer Science, vol. 91, pages 919-926; 2016.
- Sukor A.S.; Zakaria A. & Rahim N. Activity Recognition Using Accelerometer Sensor and Machine Learning Classifiers. 2018 IEEE 14th International Colloquium on Signal Processing & Its Applications (CSPA); 2018.
- Botín-Sanabria D.M.; Lozoya-Reyes J.G.; Vargas-Maldonado R.C.; Rodríguez -Hernández K.L.; Ramírez-Mendoza R.A.; Ramírez-Moreno M.A. & Lozoya-Santos J.J. Digital Tiwn for Urban Spaces: an Application. Proceedings of the International Conference on Industrial Engineering and Operations Management, Monterrey, Mexico; 2021.
- Urbanowics R.; Meeker M.; La Cava W.; Olson R. & Moore J. Relief-based feature selection: Introduction and review. Journal of Biomedical Information; 2018.