Cabrera Garcia, Jesus | Tecnológico Nacional de México, Tuxtla Gutiérrez |
Zepeda-Hernández, José Angel | Tecnológico Nacional de México, Tuxtla Gutiérrez |
López-Pérez, Manuel de Jesús | Tecnológico Nacional de México, Tuxtla Gutiérrez |
Domínguez Zenteno, Joaquín Eduardo | Tecnológico Nacional de México, Tuxtla Gutiérrez |
https://doi.org/10.58571/CNCA.AMCA.2024.091
Resumen: Estimating the State Of Health (SOH) of Lithium-Ion Batteries (LIB) is essential for optimal performance in applications such as electric vehicles (EV) and energy storage systems. However, this battery’s electrochemical parameter is impossible to measure directly. Many works have addressed estimating the battery SOH using different techniques. Recently, techniques such as machine learning have enabled data-driven predictions when a parameter cannot be measured. In this work, an artificial neuronal network (ANN) is proposed to estimate the battery’s SOH for an EV. The ANN was trained at the beginning with Ames PCoE battery data provided by NASA and complemented with synthetic data from a LIB electrochemical model. The electrochemical model describes the battery behavior with specific SOH. Therefore, a parameter estimation algorithm such as Particle Swarm Optimization (PSO) was used to figure out the model parameters for each SOH to generate new profiles and add more information to train the ANN. Finally, the ANN was implemented with a candidate battery to demonstrate its effectiveness and performance in out-range LIB operating conditions in which it was trained.
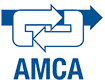
¿Cómo citar?
Cabrera Garcia, J., Zepeda Hernandez, J.A., López Pérez, M.J. & Domínguez Zenteno, J.E. (2024). SOH Estimation of Lithium-Ion Batteries Based on ANNs and Synthetic Data. Memorias del Congreso Nacional de Control Automático 2024, pp. 536-541. https://doi.org/10.58571/CNCA.AMCA.2024.091
Palabras clave
SOH, ANN, EV, Battery Model, PSO
Referencias
- Benavides Flores, M.I. (2018). Determinación de indicadores de estado de salud de baterías de ion-litio, mediante el uso de espectroscopía de impedancia electroquímica y caracterización del término de la vida útil basado en la generación de calor dada por la impedancia interna.
- Campos-Taberner, M., García-Haro, F.J., Martínez, B., and Gilabert, M. (2020). Deep learning para la clasificación de usos de suelo agrícola con sentinel-2. Revista de Teledetección, (56), 35–48.
- Chen, T., Jin, Y., Lv, H., Yang, A., Liu, M., Chen, B., Xie, Y., and Chen, Q. (2020). Applications of lithium-ion batteries in grid-scale energy storage systems. Transactions of Tianjin University, 26(3), 208–217.
- Driscoll, L., de la Torre, S., and Gomez-Ruiz, J.A. (2022). Feature-based lithium-ion battery state of health estimation with artificial neural networks. Journal of Energy Storage, 50, 104584.
- Huang, S.C., Tseng, K.H., Liang, J.W., Chang, C.L., and Pecht, M.G. (2017). An online soc and soh estimation model for lithium-ion batteries. Energies, 10(4), 512.
- Juantorena Polo, A. (2023). Estudio de la degradación de las baterías de ion-litio (lib). NA.
Li, P., Zhang, Z., Xiong, Q., Ding, B., Hou, J., Luo, D., Rong, Y., and Li, S. (2020). State-of-health estimation and remaining useful life prediction for the lithium-ion battery based on a variant long short term memory neural network. Journal of power sources, 459, 228069. - Plakhtii, O., Nerubatskyi, V., Mashura, A., and Hordiienko, D. (2020). The analysis of mathematical models of charge-discharge characteristics in lithium-ion batteries. In 2020 IEEE 40th International Conference on Electronics and Nanotechnology (ELNANO), 635–640. IEEE.
- Saha, B. and Goebel, K. (2007). Battery data set. NASA AMES prognostics data repository. Song, S. (2019). Nasa. doi:10.21227/fhxc-ga72. URL https://dx.doi.org/10.21227/fhxc-ga72.
- Torfi, A., Fox, E.A., and Reddy, C.K. (2022). Differentially private synthetic medical data generation using convolutional gans. Information Sciences, 586, 485– 500.
- Wang, D., Tan, D., and Liu, L. (2018). Particle swarm optimization algorithm: an overview. Soft computing, 22(2), 387–408.
- Zepeda H, J., Aguilar C, A., Hernández S, A., and Salgado G, M. (2015). Metodología para determinar la topología, patrones de parámetros eléctricos y. Revista Tecnología Digital, 103–113.
- Zhang, D., Zhao, W., Wang, L., Chang, X., Li, X., and Wu, P. (2022). Evaluation of the state of health of lithium-ion battery based on temporal convolution network. Frontiers in Energy Research, 761.
- Zhu, F. and Fu, J. (2021). A novel state-of-health estimation for lithium-ion battery via unscented kalman filter and improved unscented particle filter. IEEE Sensors Journal, 21(22), 25449–25456.