Gamboa Rodriguez, Edgar Arturo | Universidad Nacional Autonoma de Mexico |
Verde Rodarte, Cristina | Universidad Nacional Autonoma de Mexico |
https://doi.org/10.58571/CNCA.AMCA.2023.097
Resumen: In this work, the usage of bio-inspired algorithms for developing models capable of identifying the ubication of the anomaly, specifically a shunt fault in High Voltage Alternate Current (HVAC) for a monophase transmission line. The approach is data-driven based, where the line is simulated under different operation conditions, afterward, the cross-correlation is used for extracting the most representative characteristics of the signals. The specifications under which the genetic algorithm searched for a solution, only with the correlations, generating mathematical models are able of identifying the distance of the fault even for changing conditions. Finally, the model found by the genetic program is validated through two different sets of data (training and test), having different combinations of parameters and measuring the average error between the predicted and the real distance.
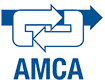
¿Cómo citar?
Gamboa Rodriguez, Edgar Arturo; Verde, Cristina. Genetic Programming for Shunt Failure Detection in Monophasic Transmission Line. Memorias del Congreso Nacional de Control Automático, pp. 591-596, 2023. https://doi.org/10.58571/CNCA.AMCA.2023.097
Palabras clave
Detección y Aislamiento de Fallas; Otros Tópicos Afines; Modelado e Identificación de Sistemas
Referencias
- Gönen, T. (2015). Electrical Power Transmission System Engineering: Analysis and Design, Third Edition. doi:10.1201/b17055.
- Indulkar, C.S. and Ramalingam, K. (2008). Estimation of transmission line parameters from measurements. International Journal of Electrical Power and Energy Systems, 30. doi:10.1016/j.ijepes.2007.08.003.
- Jamil, M., Kalam, A., Ansari, A., and Rizwan, M. (2014). Generalized neural network and wavelet transform based approach for fault location estimation of a transmission line. Applied Soft Computing, 19, 322–332. doi:https://doi.org/10.1016/j.asoc.2014.02.020. URL https://www.sciencedirect.com/science/article/pii/S1568494614000908.
- Koza, J.R. (1994). Genetic programming as a means for programming computers by natural selection. Statistics and Computing, 4. doi:10.1007/BF00175355.
- Kunj, T., Ansari, M.A., and Vishwakarrma, C.B. (2019). Transmission line fault detection and classification by using wavelet multiresolutionanalysis: A review. 2018 International Conference on Power Energy, Environment and Intelligent Control, PEEIC 2018. doi:10.1109/PEEIC.2018.8665477.
- Manohar, M., Pallavi, R., and kumar, R.P. (2016). A universal high impedance fault detection technique for distribution system using stransform and pattern recognition. Technology and Economics of Smart Grids and Sustainable Energy, 1, 9. doi:10.1007/s40866-016-0011-4. URL https://doi.org/10.1007/s40866-016-0011-4.
- Stoica, P. and Moses, R.L. (2005). Spectral analysis of signals. Pearson/Prentice Hall.
- Tekli, L., Filipovi-Gri, B., and Pavii, I. (2013). Artificial neural network approach for locating faults in power transmission system. EuroCon. doi:10.1109/eurocon.2013.6625165.
- Valdez, F., Castillo, O., and Melin, P. (2021). Bioinspired algorithms and its applications for optimization in fuzzy clustering. Algorithms, 14. doi:10.3390/a14040122.
- Verde, C., Gentil, S., and Morales-Menendez, R. (2012). Monitoreo y Diagnóstico Automático de Fallas en Sistemas Dinámicos.
- Yu, J. and Zhang, Y. (2023). Challenges and opportunities of deep learning-based process fault detection and diagnosis: a review. Neural Computing and Applications, 35. doi:10.1007/s00521-022-08017-3.
- Zhang, L., Jack, L.B., and Nandi, A.K. (2005). Fault detection using genetic programming. Mechanical Systems and Signal Processing, 19. doi:10.1016/j.ymssp.2004.03.002.